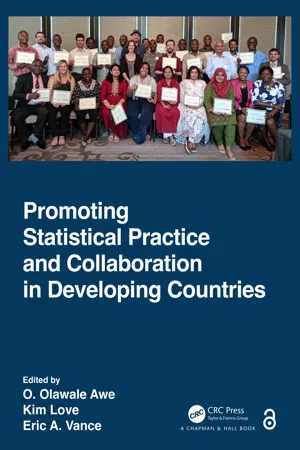
Promoting Statistical Practice and Collaboration in Developing Countries
O. Olawale Awe, Kim Love, Eric A. Vance, O. Olawale Awe, Kim Love, Eric A. Vance
- 610 pages
- English
- ePUB (mobile friendly)
- Available on iOS & Android
Promoting Statistical Practice and Collaboration in Developing Countries
O. Olawale Awe, Kim Love, Eric A. Vance, O. Olawale Awe, Kim Love, Eric A. Vance
About This Book
"Rarely, but just often enough to rebuild hope, something happens to confound my pessimism about the recent unprecedented happenings in the world. This book is the most recent instance, and I think that all its readers will join me in rejoicing at the good it seeks to do. It is an example of the kind of international comity and collaboration that we could and should undertake to solve various societal problems.
This book is a beautiful example of the power of the possible. [It] provides a blueprint for how the LISA 2020 model can be replicated in other fields. Civil engineers, or accountants, or nurses, or any other profession could follow this outline to share expertise and build capacity and promote progress in other countries. It also contains some tutorials for statistical literacy across several fields. The details would change, of course, but ideas are durable, and the generalizations seem pretty straightforward. This book shows every other profession where and how to stand in order to move the world. I urge every researcher to get a copy!"
â David Banks from the Foreword
Promoting Statistical Practice and Collaboration in Developing Countries provides new insights into the current issues and opportunities in international statistics education, statistical consulting, and collaboration, particularly in developing countries around the world. The book addresses the topics discussed in individual chapters from the perspectives of the historical context, the present state, and future directions of statistical training and practice, so that readers may fully understand the challenges and opportunities in the field of statistics and data science, especially in developing countries.
Features
⢠Reference point on statistical practice in developing countries for researchers, scholars, students, and practitioners
⢠Comprehensive source of state-of-the-art knowledge on creating statistical collaboration laboratories within the field of data science and statistics
⢠Collection of innovative statistical teaching and learning techniques in developing countries
Each chapter consists of independent case study contributions on a particular theme that are developed with a common structure and format. The common goal across the chapters is to enhance the exchange of diverse educational and action-oriented information among our intended audiences, which include practitioners, researchers, students, and statistics educators in developing countries.
Frequently asked questions
Information
Part 1 Statistics Collaboration and Practice in Developing Countries Experiences, Challenges, and Opportunities
1 Statistics and Data Science Collaboration Laboratories: Engines for Development
CONTENTS
- 1.1 Introduction: Why Statistics and Data Science Have Extraordinary Potential for Data-Driven Development
- 1.2 Collaborative Statisticians and Data Scientists
- 1.3 Statistics and Data Science Collaboration Laboratories (âStat Labsâ)
- 1.3.1 The Purpose of Stat Labs
- 1.3.2 What Stat Labs Do
- 1.3.2.1 Supporting Domain Experts
- 1.3.2.2 Creating New Knowledge
- 1.3.2.3 Transforming Evidence into Action (TEA)
- 1.3.2.4 Training the Next Generation of Collaborative Statisticians and Data Scientists
- 1.3.3 Stat Labs Produce Collaborative Statisticians and Data Scientists and Data-Capable Development Actors
- 1.4 Exemplar Stat Labs
- 1.4.1 The Laboratory for Interdisciplinary Statistical Analysis (LISA) at Virginia Tech
- 1.4.2 LISA at the University of Colorado Boulder
- 1.4.3 The University of Ibadan LISA (UI-LISA)
- 1.5 Stat Labs Can Become Engines for Development
- 1.5.1 Theory of Change
- 1.5.2 Benefits and Impacts of a Stat Lab
- 1.6 Seven Steps for Creating a Stat Lab
- 1.7 What Makes a Stat Lab Strong and Sustainable
- 1.8 Conclusion
- References
1.1 Introduction: Why Statistics and Data Science Have Extraordinary Potential for Data-Driven Development
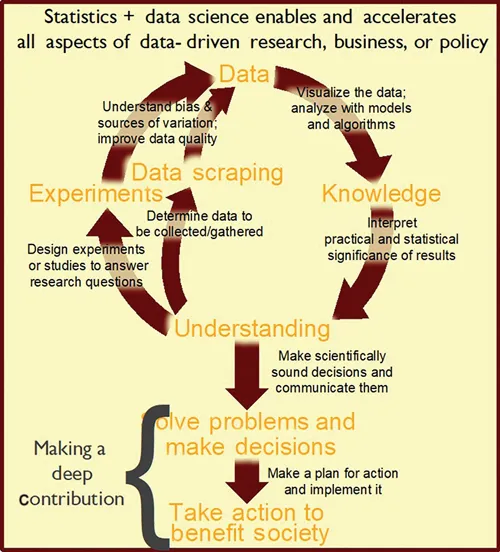
1.2 Collaborative Statisticians and Data Scientists
someone possessing deep technical skills in the theory and methods of statistics or data science and effective collaboration skills who can move between theory and practice to work with domain experts to create solutions to research, business, and policy challenges and achieve research, business, and policy goals.
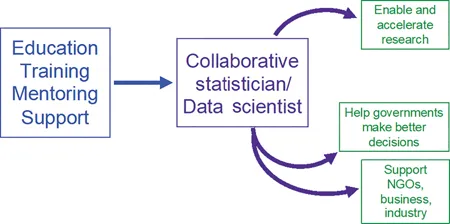
1.3 Statistics and Data Science Collaboration Laboratories (âStat Labsâ)
1.3.1 The Purpose of Stat Labs
Table of contents
- Cover
- Half Title Page
- Title Page
- Copyright Page
- Table of Contents
- Foreword
- Preface
- Editors
- Reviewers
- Contributors
- Part 1 Statistics Collaboration and Practice in Developing Countries: Experiences, Challenges, and Opportunities
- Part 2 Building Capacity in Statistical Consulting and Collaboration Techniques through the Creation of Stat Labs
- Part 3 Statistics Education and Womenâs Empowerment
- Part 4 Statistical Literacy and Methods across Disciplines
- Part 5 New Approaches to Statistical Learning in Developing Countries
- Part 6 Importance of Statistics in Urban Planning and Development
- Part 7 Statistical Literacy in the Wider Society
- Bonus Chapter: Systematically Improving Your Collaboration Practice in the 21st Century
- Index