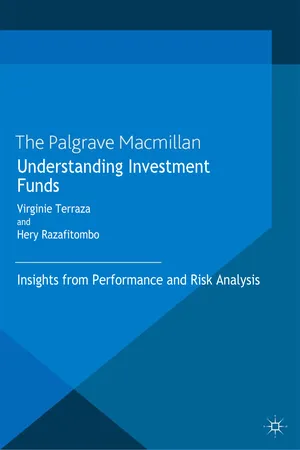
eBook - ePub
Understanding Investment Funds
Insights from Performance and Risk Analysis
This is a test
- English
- ePUB (mobile friendly)
- Available on iOS & Android
eBook - ePub
Understanding Investment Funds
Insights from Performance and Risk Analysis
Book details
Book preview
Table of contents
Citations
About This Book
In light of recent financial crises, the role of investment funds is a recurring subject for discussion. Traditional methods must be adapted with the objective to strengthen scientific knowledge of investment funds. This book provides new insights, ideas and empirical evidence to improve tools and methods for fund performance analysis.
Frequently asked questions
At the moment all of our mobile-responsive ePub books are available to download via the app. Most of our PDFs are also available to download and we're working on making the final remaining ones downloadable now. Learn more here.
Both plans give you full access to the library and all of Perlegoâs features. The only differences are the price and subscription period: With the annual plan youâll save around 30% compared to 12 months on the monthly plan.
We are an online textbook subscription service, where you can get access to an entire online library for less than the price of a single book per month. With over 1 million books across 1000+ topics, weâve got you covered! Learn more here.
Look out for the read-aloud symbol on your next book to see if you can listen to it. The read-aloud tool reads text aloud for you, highlighting the text as it is being read. You can pause it, speed it up and slow it down. Learn more here.
Yes, you can access Understanding Investment Funds by V. Terraza, H. Razafitombo, V. Terraza,H. Razafitombo in PDF and/or ePUB format, as well as other popular books in Business & Financial Risk Management. We have over one million books available in our catalogue for you to explore.
Information
Part I
New Performance Measure Methodologies
1
Is There a Link between Past Performance and Fund Failure?
Philippe Cogneau, Laurent Bodson, and Georges HĂźbner
1.1 Introduction
Mutual fund survivorship has been an ongoing concern since the early 1990s for different reasons. Many researchers have studied this phenomenon because of the so-called survivorship bias. Indeed, ignoring the funds that disappear while analyzing the performance of funds generates an important bias â since the funds that failed during the period are omitted, only the funds that were alive during the whole period are selected. Another stream of papers has focused on the assessment of the percentage of âgraveyardâ funds, that is, those that disappear within a certain period. Fewer have aimed to examine the determinants of the fund terminations.
In their study dedicated to the determinants of mutual funds survivorship, Brown and Goetzmann (1995) show that the likelihood of fund disappearance is linked to past returns, going back three years. Carhart (1997) even finds that dead funds underperform for up to five years before their disappearance. Other well-known analysis in this context, showing that only best performers survive for a long period of time, while weaker are likely to be closed, are due to Brown et al. (1992), Malkiel (1995), and Elton et al. (1996). Cameron and Hall (2003) show that relative returns (defined as the excess to a market index) are much better predictors of fund failure than gross returns. They find an asymmetric link between shocks and disappearance: positive shocks have a larger impact than negative shocks.
In parallel, some researchers (Sawicki, 2001; Sirri and Tufano, 1998) show that investors base their fund purchase decisions on prior performance. However, in most studies following this approach, most of the authors solely focus on classical performance measures: gross return, return on excess of a market index, Jensen alpha (Jensen, 1968), Fama and French 3-factors alpha (Fama and French, 1993, Carhart 4-factors alpha (Carhart, 1997). Rohleder et al. (2011) compare the results given by the last four different measures to estimate the size of the survivorship bias obtained with different methods in US mutual funds data.
In the last decade, the research on mutual fund survival has largely diverged from the study of past performance. Many other determinants of fund death have been investigated: size (Brown and Goetzmann, 1995; Carhart et al., 2002), age (Brown and Goetzmann, 1995; Lunde et al., 1999), style (Horst et al., 2001; Bu and Lacey, 2009, expense ratios (Carhart et al., 2002; Bu and Lacey, 2009 or incentives (Massa and Patgiri (2009)), among others. The interest in prior performance and risk as predictors of fund failure has moved to the hedge funds literature. In a recent analysis, Liang and Park (2010) consider different risk measures to adjust performance. They show that semi-deviation, VaR, conditional VaR (CVaR), expected shortfall and tail risk are better predictors than standard deviation (especially the latter two). Other studies, such as Chapman et al. (2008) and Ng (2008), have developed models capable of forecasting hedge fund failure. They use the same performance measures mentioned in the mutual fund analysis.
In this chapter, we get back to the intuition that past performance would naturally stand as a primary determinant of the decision to shut down a mutual fund. At the same time, we acknowledge that the literature on performance measurement has considerably evolved since the seminal studies in the field,1 and wish to take advantage of this progress. Our study aims at systematically investigating the drivers of past performance and detecting whether a multi-dimensional representation of a fundâs performance could be helpful in predicting its survival.
To the best of our knowledge, ours is the first paper dedicated to a comprehensive analysis of the predictive properties of performance measures for fund survival. Our choice of focusing on forecasting the probability of survivorship rather than on persistence in performance is motivated by a hierarchical concern. For an investor, it is much more important to be able to anticipate a fundâs death than to be able to pick superior future performers, because the consequences of making the wrong bet are far more penalizing in the first case.
While many researchers apply the Cox proportional hazards model to forecast the fundsâ survival time, our focus is on the likelihood of a fundâs disappearance within a certain period. We thus consider a logit approach to link a fundâs disappearance to its past performance determinants. We also introduce two extensions in the analysis, by using weekly data and considering different international fund markets, when most of the research is concentrated on US data or other national markets (Australia for Cameron and Hall, 2003 and Sawicki, 2001, and the United Kingdom for Lunde et al., 1999). Finally, we also distinguish the reason for a fundâs disappearance and examine whether differential effects can be emphasized.
1.2 Data and variable construction
We start with the description of the data sets used for the analysis and of their treatment in order to be workable in the scope of the study. Next, we describe the methods used for the construction of variables used as predictors of fund failure.
1.2.1 Data
Mutual fund data
We handle a database of weekly returns of 11,342 mutual funds with major or full allocation in equities on a worldwide basis. The time window ranges from Friday 30 December 1994 to Friday 8 January 2010, so 15 years of data. Our data source is the CRSP Survivor Bias Free Mutual Fund Database.2 We avoid the issue of the distribution of dividends, which may have a tax impact for investors in different countries, by restricting the sample to only accumulation funds. With information on the fund type, retrieved in Bloomberg, the sample is shrunk to 2,643 funds.
Elton et al. (2001) emphasize that the CRSP mutual fund database, although it is comprehensive and exempt from survivorship bias, might exhibit problems of redundant data for some funds, possibly impacting their betas and alphas, and in turn affecting other performance metrics. As this kind of bias would strongly influence our results, we take strong action to mitigate it. Namely, we exclude from the sample all funds for which the holes in the series of weekly prices are potentially suspicious. We throw away all funds having at least three times three consecutive identical prices or at least eight times two consecutive identical prices.
Finally, to get workable homogeneity in the asset pricing specifications used to compute many of the performance measures, we keep only the funds in the most important currency. This leaves us with a final sample of 1614 funds: 675 in EUR, 679 in GBP, 228 in USD and 32 in JPY.3 Summary statistics about these 1,614 funds is given in Table 1.1.
Table 1.1 Summary statistics of the fund returns
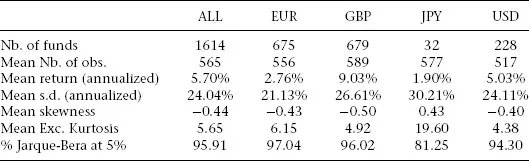
Even though the sample period encompasses the 2007â2008 crisis, the yearly returns are positive on average and their standard deviations are in the neighborhood of 20 to 30 percent, for all currencies. Skewness is negative excepted for the funds in JPY, and kurtosis is very positive, especially for funds in JPY.
The last row indicates the frequency at which the hypothesis of norma...
Table of contents
- Cover
- Title Page
- Copyright
- Contents
- List of Figures
- List of Tables
- Acknowledgments
- Notes on Contributors
- Introduction
- Part I New Performance Measure Methodologies
- Part II Advanced Risk Analyses and Modeling
- Index