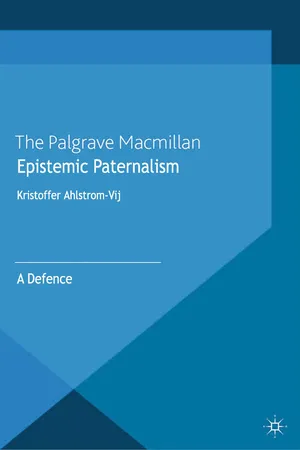
This is a test
- English
- ePUB (mobile friendly)
- Available on iOS & Android
eBook - ePub
Book details
Book preview
Table of contents
Citations
About This Book
Any attempt to help us reason in more accurate ways faces a problem: While we acknowledge that others stand to benefit from intellectual advice, each and every one of us tends to consider ourselves an exception, on account of overconfidence. The solution? Accept a form of epistemic paternalism.
Frequently asked questions
At the moment all of our mobile-responsive ePub books are available to download via the app. Most of our PDFs are also available to download and we're working on making the final remaining ones downloadable now. Learn more here.
Both plans give you full access to the library and all of Perlegoâs features. The only differences are the price and subscription period: With the annual plan youâll save around 30% compared to 12 months on the monthly plan.
We are an online textbook subscription service, where you can get access to an entire online library for less than the price of a single book per month. With over 1 million books across 1000+ topics, weâve got you covered! Learn more here.
Look out for the read-aloud symbol on your next book to see if you can listen to it. The read-aloud tool reads text aloud for you, highlighting the text as it is being read. You can pause it, speed it up and slow it down. Learn more here.
Yes, you can access Epistemic Paternalism by Kenneth A. Loparo in PDF and/or ePUB format, as well as other popular books in Philosophy & Political Philosophy. We have over one million books available in our catalogue for you to explore.
Information
Topic
PhilosophySubtopic
Political Philosophy1
Why We Cannot Rely on Ourselves for Epistemic Improvement
1.1 The pitfalls of heuristical reasoning
Largely due to the seminal work of cognitive psychologists Amos Tversky and Daniel Kahneman, it is now well known that we have certain systematic and predictable tendencies to reason in ways that lead us to form inaccurate beliefs.1 While probably well-worn territory for some philosophers, dwelling for a moment on exactly what does and does not follow from their studies, as well as from the many studies by other psychologists working on questions spawned by Tversky and Kahnemanâs research, will make clear what is at stake for the present investigation, as it relates to the relevant empirical research. That said, those familiar with the relevant research, and on board with the idea that it gives us reason to think about how to develop ameliorative strategies in response to it, may skip ahead to Section 1.4.
Let us start by considering some examples of the kind of cognitive tendencies at issue. One much discussed tendency that Tversky and Kahneman identified was that, when reasoning about the likelihood or prevalence of a certain outcome, we tend to rely heavily â indeed, sometimes too heavily â on information that happens to be readily available. For example, when I try to assess the divorce rate in my community, I might do so by recalling how many of my friends and acquaintances have obtained a divorce. Similarly, when I try to estimate the prevalence of cocaine use in Hollywood, I might do this by calling to mind vivid instances of cocaine using Hollywood celebrities. When making estimations by the ease with which particular instances or associations can be brought to mind in this manner, I am relying on what Tversky and Kahneman refer to as the availability heuristic.2
In many situations, such heuristical reasoning â roughly, reasoning that proceeds by way of sub-personal rules of thumb that operate on a limited number of cues, rather than through the systematic application of formal rules or principles of logic, statistics and probability theory â can lead to perfectly accurate results. The problem with relying too heavily on the availability heuristic, however, is that the ease with which we are able to bring certain kinds of information to mind often has little to do with whether or not that information constitutes good or probative information. This becomes particularly obvious when considering how cognitive availability often has more to do with the relevant scenarios tugging at our emotional strings than with those scenarios occurring with any great frequency. Tversky and Kahneman illustrate the point as follows:
Many readers must have experienced the temporary rise in the subjective probability of an accident after seeing a car overturned by the side of the road. Similarly, many must have noticed an increase in the subjective probability that an accident or malfunction will start a thermonuclear war after seeing a movie in which such an occurrence was vividly portrayed. Continued preoccupation with an outcome may increase its availability, and hence its perceived likelihood. People are preoccupied with highly desirable outcomes, such as winning the sweepstakes, or with highly undesirable outcomes, such as an airplane crash. Consequently, availability provides a mechanism by which occurrences of extreme utility (or disutility) may appear more likely than they actually are.3
Another heuristic that has received a lot of attention in the literature is the so-called representativeness heuristic. When reasoning by way of the representativeness heuristics, we are assuming that any given sample will be representative of the population from which it is drawn, even if the sample in question is very small. On account of this heuristic, we tend to happily project properties onto populations based on very small samples, thereby flouting the statisticianâs law of large numbers, according to which the properties of the population can be expected to resemble those of the sample only when the sample is large. As Tversky and Kahneman point out, peopleâs intuitions about random sampling thereby âappear to satisfy the law of small numbers, which asserts that the law of large numbers applies to small numbers as wellâ.4
One consequence of relying too heavily on representativeness is regression neglect. Regression neglect arises in situations where two sequential observations of the value of a variable (for example, the performance on a particular test) are radically different. Given our tendency to generalize even from small samples (in this case, from the first observation to the second), the radical difference between the two observations presents a prima facie problem for the generalizing subject. Regression neglect occurs when we account for this discrepancy by invoking an incorrect causal explanation of why the two observations differ, even when the best explanation of the discrepancy is one of regression towards the mean, that is, the tendency of the values of imperfect predictors to move towards the mean over time.5 Kahneman, Tversky and Paul Slovic nicely illustrate the practical â and potentially detrimental â influence of regression neglect on our judgments:
In a discussion of flight training, experienced instructors noted that praise for an exceptionally smooth landing is typically followed by a poorer landing on the next try, while harsh criticism after a rough landing is usually followed by an improvement on the next try. The instructors concluded that verbal rewards are detrimental to learning, while verbal punishments are beneficial, contrary to accepted psychological doctrine. This conclusion is unwarranted because of the presence of regression toward the mean. As in other cases of repeated examination, an improvement will usually follow a poor performance and a deterioration will usually follow an outstanding performance, even if the instructor does not respond to the traineeâs achievement on the first attempt. Because the instructors had praised their trainees after good landings and admonished them after poor ones, they reached the erroneous and potentially harmful conclusion that punishment is more effective than reward.6
By the same token, any public policy intervention aimed at an unusual characteristic or a group that is very different from the average is likely to appear successful, whereas success often is nothing but an instance of regression to the mean. This may happen for example in public health interventions, which are often aimed at sudden increases in disease. Analogously, the phenomenon can lead to misinterpretation of the results of tests, as well as to a placebo effect in clinical studies, especially if participants in the studies are recruited on the basis of scoring highly on a symptom index.
Further research suggests that we also have a strong tendency to be anchored by readily available pieces of information in our numerical estimations â information that might or might not be relevant, and that we subsequently often fail to correct for in our final estimate.7 For example, our risk assessments are heavily influenced by the first piece of data that we are provided with, and as such vary depending on whether we are first presented with, say, the 50,000 annual deaths from motor vehicle accidents or with the 1,000 annual deaths from electrocution.8 The same goes for our price estimations, even in cases where we are provided with preposterously extreme anchor values, such as âIs the average price of a college textbook more or less than $7,128?â9 But the phenomenon of anchoring is perhaps most vividly illustrated by how it manifests itself in civil tort lawsuit situations, where the amount of money requested by the plaintiff has been shown to anchor mock juriesâ decisions to such an extent that the researchers studying them titled their report âThe More You Ask For, the More You Getâ.10
1.2 Why not to equate heuristics with biases
So far, I have stayed clear of a term that many might have expected should have cropped up by now. That term is âbiasâ. In the psychological literature, biases are sometimes identified with any instance of heuristical reasoning that flouts principles gleaned from logic, statistics and probability theory in a systematic manner.11 However, we should take care not to confuse heuristical reasoning â again, reasoning that proceeds by way of sub-personal rules of thumb rather than through the systematic application of formal rules or principles of logic, statistics and probability theory â with biased reasoning. Granted, the previous reasoning patterns could all be categorized as heuristical, but that is not what renders them (or rather certain instances of them) biases. Whether or not an instance of heuristical reasoning amounts to a bias depends not only on the nature of the heuristic involved but also on the context in which it is applied.
To illustrate this point, let us return to the law of small numbers. Does our tendency to reason in accordance with this âlawâ always constitute a bias? According to Hilary Kornblith, it is not clear that it does.12 Suppose, for example, that you and I have to predict whether the next ball drawn from an urn will be black or white. You examine each ball in the urn and predict that the next ball to be drawn will be of whatever turns out to be the preponderant colour. On the other hand, I simply sample one ball, and predict that the next ball to be drawn will be of the same colour. Who is most likely to be right? That depends on the distribution of colours in the urn, of course. Therefore, let us assume that there are 90 per cent white balls and 10 per cent black balls in the urn. In that case, you will be right 90 per cent of the time, and I will be right 82 per cent ((0.9 x 0.9) + (0.1 x 0.1)) of the time. Not a huge difference, in other words. Now, assume that the colour proportions are even. In that case, we will both be right 50 per cent of the time â despite you investing a great deal more effort into determining the proportions in question. Kornblith concludes:
The fact is that when predictions are made about a single case, prediction based on the law of small numbers is not very much inferior to the best statistical methods. Indeed, from a practical point of view, use of the law of small numbers may frequently be preferred. If the cost of gaining additional information is high, the tradeoff of reliability for ease of information gathering may be altogether reasonable, especially given the small loss of reliability and the possibility of having to search a very large sample space. In the case of making a single prediction about the population, beliefs based on the law of small numbers are thus nearly as accurate as any of the available alternatives.13
Kornblith is of course not trying to make a case for giving up on the law of large numbers in statistical inference. The point is simply that something being a bias is not something that simply can be read off the fact that it violates some statistical principle. As Kornblithâs example makes clear, there are situations in which reasoning by way of heuristics that violate statistical principles will sometimes not make for a significant reduction (if any) in an agentâs reliability, and may even be preferable on practical grounds to more labour-intensive strategies.
Kornblith calls our attention to cases in which we are not significantly worse off epistemically for engaging in heuristical reasoning, and it for that reason does not seem appropriate to describe the relevant instances of such reasoning as being biased. Might there even be cases wherein we are better off for reasoning heuristically? According to Gerd Gigerenzer and Daniel Goldstein, it seems that there is.14 Gigerenzer and Goldstein posed the following question to a group of US students and to a group of German students: which city has more inhabitants, San Diego or San Antonio? Sixty-two per cent of the US students were able to answer the question correctly (San Diego). Surprisingly, however, all of the German students gave the correct answer. In order to rule out that this was a mere artefact of Germans knowing...
Table of contents
- Cover
- Title
- Introduction
- 1Â Â Why We Cannot Rely on Ourselves for Epistemic Improvement
- 2Â Â Epistemic Paternalism Defined
- 3Â Â On the Viability of Epistemic Paternalism: Personal Autonomy
- 4Â Â On the Viability of Epistemic Paternalism: Epistemic Autonomy
- 5Â Â Justifying Epistemic Paternalism
- 6Â Â Epistemic Paternalism Defended
- Bibliography
- Index