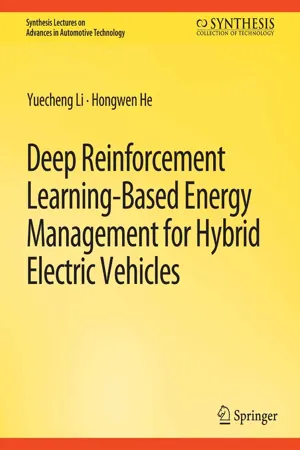
Deep Reinforcement Learning-based Energy Management for Hybrid Electric Vehicles
- English
- PDF
- Available on iOS & Android
Deep Reinforcement Learning-based Energy Management for Hybrid Electric Vehicles
About This Book
The urgent need for vehicle electrification and improvement in fuel efficiency has gained increasing attention worldwide. Regarding this concern, the solution of hybrid vehicle systems has proven its value from academic research and industry applications, where energy management plays a key role in taking full advantage of hybrid electric vehicles (HEVs). There are many well-established energy management approaches, ranging from rules-based strategies to optimization-based methods, that can provide diverse options to achieve higher fuel economy performance. However, the research scope for energy management is still expanding with the development of intelligent transportation systems and the improvement in onboard sensing and computing resources. Owing to the boom in machine learning, especially deep learning and deep reinforcement learning (DRL), research on learning-based energy management strategies (EMSs) is gradually gaining more momentum. They have shown great promise in not onlybeing capable of dealing with big data, but also in generalizing previously learned rules to new scenarios without complex manually tunning. Focusing on learning-based energy management with DRL as the core, this book begins with an introduction to the background of DRL in HEV energy management. The strengths and limitations of typical DRL-based EMSs are identified according to the types of state space and action space in energy management. Accordingly, value-based, policy gradient-based, and hybrid action space-oriented energy management methods via DRL are discussed, respectively. Finally, a general online integration scheme for DRL-based EMS is described to bridge the gap between strategy learning in the simulator and strategy deployment on the vehicle controller.
Frequently asked questions
Information
Table of contents
- Cover
- Copyright Page
- Title Page
- Contents
- Introduction
- Background: Deep Reinforcement Learning
- Learning of EMSs in Continuous State SpaceâDiscrete Action Space
- Learning of EMSs in Continuous StateâContinuous Action Space
- Learning of EMSs in Discrete-Continuous Hybrid Action Space
- An Online Integration Scheme for DRL-Based EMSs
- Conclusions
- Bibliography
- Authorsâ Biographies