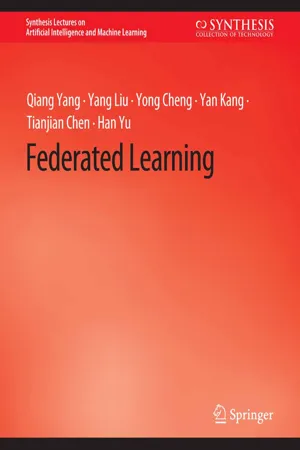
Federated Learning
Qiang Yang, Yang Liu, Yong Cheng, Yan Kang, Tianjian Chen, Han Yu
- English
- PDF
- Available on iOS & Android
Federated Learning
Qiang Yang, Yang Liu, Yong Cheng, Yan Kang, Tianjian Chen, Han Yu
About This Book
How is it possible to allow multiple data owners to collaboratively train and use a shared prediction model while keeping all the local training data private?
Traditional machine learning approaches need to combine all data at one location, typically a data center, which may very well violate the laws on user privacy and data confidentiality. Today, many parts of the world demand that technology companies treat user data carefully according to user-privacy laws. The European Union's General Data Protection Regulation (GDPR) is a prime example. In this book, we describe how federated machine learning addresses this problem with novel solutions combining distributed machine learning, cryptography and security, and incentive mechanism design based on economic principles and game theory. We explain different types of privacy-preserving machine learning solutions and their technological backgrounds, and highlight some representative practical use cases. We show how federated learning can become the foundation of next-generation machine learning that caters to technological and societal needs for responsible AI development and application.
Frequently asked questions
Information
Table of contents
- Cover
- Title Page
- Copyright Page
- Contents
- Preface
- Acknowledgments
- Introduction
- Background
- Distributed Machine Learning
- Horizontal Federated Learning
- Vertical Federated Learning
- Federated Transfer Learning
- Incentive Mechanism Design for Federated Learning
- Federated Learning for Vision, Language, and Recommendation
- Federated Reinforcement Learning
- Selected Applications
- Summary and Outlook
- Legal Development on Data Protection
- Bibliography
- Authors' Biographies