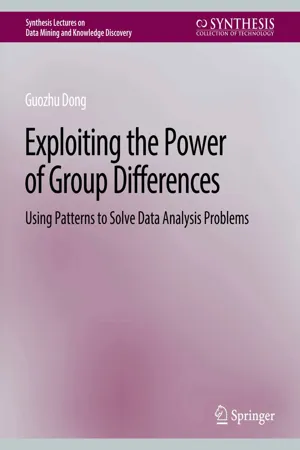
Exploiting the Power of Group Differences
Using Patterns to Solve Data Analysis Problems
- English
- PDF
- Available on iOS & Android
About This Book
This book presents pattern-based problem-solving methods for a variety of machine learning and data analysis problems. The methods are all based on techniques that exploit the power of group differences. They make use of group differences represented using emerging patterns (aka contrast patterns), which are patterns that match significantly different numbers of instances in different data groups. A large number of applications outside of the computing discipline are also included.
Emerging patterns (EPs) are useful in many ways. EPs can be used as features, as simple classifiers, as subpopulation signatures/characterizations, and as triggering conditions for alerts. EPs can be used in gene ranking for complex diseases since they capture multi-factor interactions. The length of EPs can be used to detect anomalies, outliers, and novelties. Emerging/contrast pattern based methods for clustering analysis and outlier detection do not need distance metrics, avoiding pitfalls of the latter in exploratory analysis of high dimensional data. EP-based classifiers can achieve good accuracy even when the training datasets are tiny, making them useful for exploratory compound selection in drug design. EPs can serve as opportunities in opportunity-focused boosting and are useful for constructing powerful conditional ensembles. EP-based methods often produce interpretable models and results. In general, EPs are useful for classification, clustering, outlier detection, gene ranking for complex diseases, prediction model analysis and improvement, and so on.
EPs are useful for many tasks because they represent group differences, which have extraordinary power. Moreover, EPs represent multi-factor interactions, whose effective handling is of vital importance and is a major challenge in many disciplines.
Based on the results presented in this book, one can clearly say that patterns are useful, especially when they are linked to issues of interest.
We believe that many effective ways to exploit group differences' power still remain to be discovered. Hopefully this book will inspire readers to discover such new ways, besides showing them existing ways, to solve various challenging problems.
Frequently asked questions
Information
Table of contents
- Cover
- Copyright Page
- Title Page
- Dedication
- Contents
- Acknowledgments
- Introduction and Overview
- General Preliminaries
- Emerging Patterns and a Flexible Mining Algorithm
- CAEP: Classification By Aggregating Multiple Matching Emerging Patterns
- CAEP for Classification on Tiny Training Datasets, Compound Selection, and Instance Selection
- OCLEP: One-Class Intrusion Detection and Anomaly Detection
- CPCQ: Contrast Pattern Based Clustering-Quality Evaluation
- CPC: Pattern-Based Clustering Maximizing CPCQ
- IBIG: Ranking Genes and Attributes for Complex Diseases and Complex Problems
- CPXR and CPXC: Pattern Aided Prediction Modeling and Prediction Model Analysis
- Other Approaches and Applications Using Emerging Patterns
- Bibliography
- Authorâs Biography
- Index