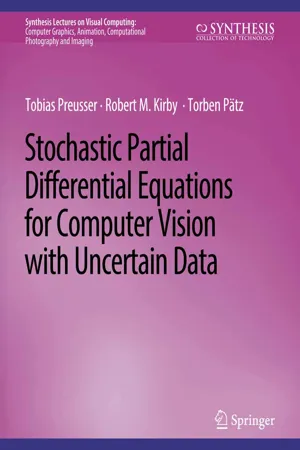
Stochastic Partial Differential Equations for Computer Vision with Uncertain Data
- English
- PDF
- Available on iOS & Android
Stochastic Partial Differential Equations for Computer Vision with Uncertain Data
About This Book
In image processing and computer vision applications such as medical or scientific image data analysis, as well as in industrial scenarios, images are used as input measurement data. It is good scientific practice that proper measurements must be equipped with error and uncertainty estimates. For many applications, not only the measured values but also their errors and uncertainties, should be—and more and more frequently are—taken into account for further processing. This error and uncertainty propagation must be done for every processing step such that the final result comes with a reliable precision estimate. The goal of this book is to introduce the reader to the recent advances from the field of uncertainty quantification and error propagation for computer vision, image processing, and image analysis that are based on partial differential equations (PDEs). It presents a concept with which error propagation and sensitivity analysis can be formulated with a set of basic operations.The approach discussed in this book has the potential for application in all areas of quantitative computer vision, image processing, and image analysis. In particular, it might help medical imaging finally become a scientific discipline that is characterized by the classical paradigms of observation, measurement, and error awareness. This book is comprised of eight chapters. After an introduction to the goals of the book (Chapter 1), we present a brief review of PDEs and their numerical treatment (Chapter 2), PDE-based image processing (Chapter 3), and the numerics of stochastic PDEs (Chapter 4). We then proceed to define the concept of stochastic images (Chapter 5), describe how to accomplish image processing and computer vision with stochastic images (Chapter 6), and demonstrate the use of these principles for accomplishing sensitivity analysis (Chapter 7). Chapter 8 concludes the book and highlights new research topics for the future.
Frequently asked questions
Information
Table of contents
- Cover
- Copyright Page
- Title Page
- Contents
- Preface
- Notation
- Introduction
- Partial Differential Equations and Their Numerics
- Review of PDE-Based Image Processing
- Numerics of Stochastic PDEs
- Stochastic Images
- Image Processing and Computer Vision with Stochastic Images
- Sensitivity Analysis
- Conclusions
- Bibliography
- Authors' Biographies