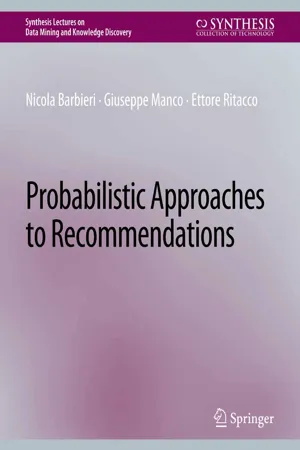
Probabilistic Approaches to Recommendations
- English
- PDF
- Available on iOS & Android
Probabilistic Approaches to Recommendations
About This Book
The importance of accurate recommender systems has been widely recognized by academia and industry, and recommendation is rapidly becoming one of the most successful applications of data mining and machine learning. Understanding and predicting the choices and preferences of users is a challenging task: real-world scenarios involve users behaving in complex situations, where prior beliefs, specific tendencies, and reciprocal influences jointly contribute to determining the preferences of users toward huge amounts of information, services, and products. Probabilistic modeling represents a robust formal mathematical framework to model these assumptions and study their effects in the recommendation process. This book starts with a brief summary of the recommendation problem and its challenges and a review of some widely used techniques Next, we introduce and discuss probabilistic approaches for modeling preference data. We focus our attention on methods based on latent factors, such as mixture models, probabilistic matrix factorization, and topic models, for explicit and implicit preference data. These methods represent a significant advance in the research and technology of recommendation. The resulting models allow us to identify complex patterns in preference data, which can be exploited to predict future purchases effectively. The extreme sparsity of preference data poses serious challenges to the modeling of user preferences, especially in the cases where few observations are available. Bayesian inference techniques elegantly address the need for regularization, and their integration with latent factor modeling helps to boost the performances of the basic techniques. We summarize the strengths and weakness of several approaches by considering two different but related evaluation perspectives, namely, rating prediction and recommendation accuracy. Furthermore, we describe how probabilistic methods based on latent factors enable the exploitation of preference patterns in novel applications beyond rating prediction or recommendation accuracy. We finally discuss the application of probabilistic techniques in two additional scenarios, characterized by the availability of side information besides preference data. In summary, the book categorizes the myriad probabilistic approaches to recommendations and provides guidelines for their adoption in real-world situations.
Frequently asked questions
Information
Table of contents
- Cover
- Copyright Page
- Title Page
- Dedication
- Contents
- Preface
- 1 The Recommendation Process
- 2 Probabilistic Models for Collaborative Filtering
- 3 Bayesian Modeling
- 4 Exploiting Probabilistic Models
- 5 Contextual Information
- 6 Social Recommender Systems
- 7 Conclusions
- A Parameter Estimation and Inference
- Bibliography
- Authorsâ Biographies