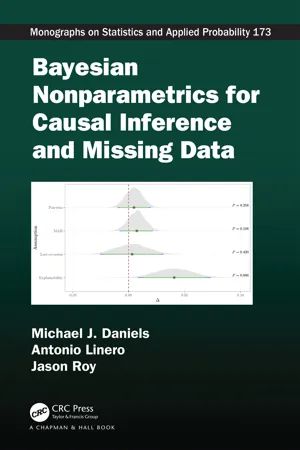
Bayesian Nonparametrics for Causal Inference and Missing Data
- 248 pages
- English
- ePUB (mobile friendly)
- Available on iOS & Android
Bayesian Nonparametrics for Causal Inference and Missing Data
About This Book
Bayesian Nonparametrics for Causal Inference and Missing Data provides an overview of flexible Bayesian nonparametric (BNP) methods for modeling joint or conditional distributions and functional relationships, and their interplay with causal inference and missing data. This book emphasizes the importance of making untestable assumptions to identify estimands of interest, such as missing at random assumption for missing data and unconfoundedness for causal inference in observational studies. Unlike parametric methods, the BNP approach can account for possible violations of assumptions and minimize concerns about model misspecification. The overall strategy is to first specify BNP models for observed data and then to specify additional uncheckable assumptions to identify estimands of interest.
The book is divided into three parts. Part I develops the key concepts in causal inference and missing data and reviews relevant concepts in Bayesian inference. Part II introduces the fundamental BNP tools required to address causal inference and missing data problems. Part III shows how the BNP approach can be applied in a variety of case studies. The datasets in the case studies come from electronic health records data, survey data, cohort studies, and randomized clinical trials.
Features
⢠Thorough discussion of both BNP and its interplay with causal inference and missing data
⢠How to use BNP and g-computation for causal inference and non-ignorable missingness
⢠How to derive and calibrate sensitivity parameters to assess sensitivity to deviations from uncheckable causal and/or missingness assumptions
⢠Detailed case studies illustrating the application of BNP methods to causal inference and missing data
⢠R code and/or packages to implement BNP in causal inference and missing data problems
The book is primarily aimed at researchers and graduate students from statistics and biostatistics. It will also serve as a useful practical reference for mathematically sophisticated epidemiologists and medical researchers.
Frequently asked questions
Information
Table of contents
- Cover Page
- Half-Title Page
- Series Page
- Title Page
- Copyright Page
- Dedication Page
- Contents
- Preface
- I Overview of Bayesian inference in causal inference and missing data and identifiability
- II Bayesian nonparametrics for causal inference and missing data
- III Case studies
- Bibliography
- Index