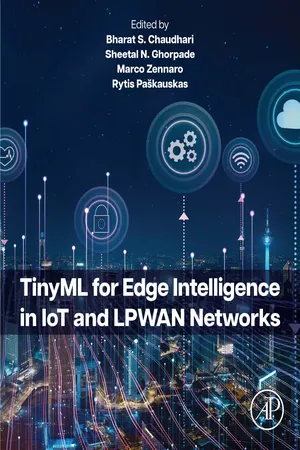
TinyML for Edge Intelligence in IoT and LPWAN Networks
Bharat S Chaudhari,Sheetal N Ghorpade,Marco Zennaro,Rytis Paškauskas
- 385 pages
- English
- ePUB (mobile friendly)
- Available on iOS & Android
TinyML for Edge Intelligence in IoT and LPWAN Networks
Bharat S Chaudhari,Sheetal N Ghorpade,Marco Zennaro,Rytis Paškauskas
About This Book
Recently, Tiny Machine Learning (TinyML) has gained incredible importance due to its capabilities of creating lightweight machine learning (ML) frameworks aiming at low latency, lower energy consumption, lower bandwidth requirement, improved data security and privacy, and other performance necessities. As billions of battery-operated embedded IoT and low power wide area networks (LPWAN) nodes with very low on-board memory and computational capabilities are getting connected to the Internet each year, there is a critical need to have a special computational framework like TinyML.
TinyML for Edge Intelligence in IoT and LPWAN Networks presents the evolution, developments, and advances in TinyML as applied to IoT and LPWANs. It starts by providing the foundations of IoT/LPWANs, low power embedded systems and hardware, the role of artificial intelligence and machine learning in communication networks in general and cloud/edge intelligence. It then presents the concepts, methods, algorithms and tools of TinyML. Practical applications of the use of TinyML are given from health and industrial fields which provide practical guidance on the design of applications and the selection of appropriate technologies.
TinyML for Edge Intelligence in IoT and LPWAN Networks is highly suitable for academic researchers and professional system engineers, architects, designers, testers, deployment engineers seeking to design ultra-lower power and time-critical applications. It would also help in designing the networks for emerging and future applications for resource-constrained nodes.
- This book provides one-stop solutions for emerging TinyML for IoT and LPWAN applications.
- The principles and methods of TinyML are explained, with a focus on how it can be used for IoT, LPWANs, and 5G applications.
- Applications from the healthcare and industrial sectors are presented.
- Guidance on the design of applications and the selection of appropriate technologies is provided.
Frequently asked questions
Information
Table of contents
- Cover image
- Title page
- Table of Contents
- Copyright
- List of contributors
- Preface
- Acknowledgments
- Chapter 1: TinyML for low-power Internet of Things
- Chapter 2: Embedded systems for low-power applications
- Chapter 3: Cloud and edge intelligence
- Chapter 4: TinyML: principles and algorithms
- Chapter 5: TinyML using neural networks for resource-constrained devices
- Chapter 6: Reinforcement learning for LoRaWANs
- Chapter 7: Software frameworks for TinyML
- Chapter 8: Extensive energy modeling for LoRaWANs
- Chapter 9: TinyML for 5G networks
- Chapter 10: Non-static TinyML for ad hoc networked devices
- Chapter 11: Bayesian-driven optimizations of TinyML for efficient edge intelligence in LPWANs
- Chapter 12: 6TiSCH adaptive scheduling for Industrial Internet of Things
- Chapter 13: Securing TinyML in a connected world
- Chapter 14: TinyML applications and use cases for healthcare
- Chapter 15: Machine learning techniques for indoor localization on edge devices
- Chapter 16: Embedded intelligence in Internet of Things scenarios: TinyML meets eBPF
- Chapter 17: A real-time price recognition system using lightweight deep neural networks on mobile devices
- Chapter 18: TinyML network applications for smart cities
- Chapter 19: Emerging application use cases and future directions
- Index