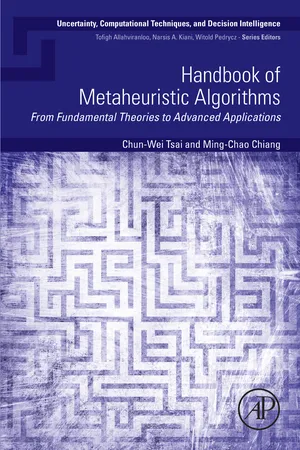
Handbook of Metaheuristic Algorithms
From Fundamental Theories to Advanced Applications
- 622 pages
- English
- ePUB (mobile friendly)
- Only available on web
Handbook of Metaheuristic Algorithms
From Fundamental Theories to Advanced Applications
About This Book
Handbook of Metaheuristic Algorithms: From Fundamental Theories to Advanced Applications provides a brief introduction to metaheuristic algorithms from the ground up, including basic ideas and advanced solutions. Although readers may be able to find source code for some metaheuristic algorithms on the Internet, the coding styles and explanations are generally quite different, and thus requiring expanded knowledge between theory and implementation. This book can also help students and researchers construct an integrated perspective of metaheuristic and unsupervised algorithms for artificial intelligence research in computer science and applied engineering domains.
Metaheuristic algorithms can be considered the epitome of unsupervised learning algorithms for the optimization of engineering and artificial intelligence problems, including simulated annealing (SA), tabu search (TS), genetic algorithm (GA), ant colony optimization (ACO), particle swarm optimization (PSO), differential evolution (DE), and others. Distinct from most supervised learning algorithms that need labeled data to learn and construct determination models, metaheuristic algorithms inherit characteristics of unsupervised learning algorithms used for solving complex engineering optimization problems without labeled data, just like self-learning, to find solutions to complex problems.
- Presents a unified framework for metaheuristics and describes well-known algorithms and their variants
- Introduces fundamentals and advanced topics for solving engineering optimization problems, e.g., scheduling problems, sensors deployment problems, and clustering problems
- Includes source code based on the unified framework for metaheuristics used as examples to show how TS, SA, GA, ACO, PSO, DE, parallel metaheuristic algorithm, hybrid metaheuristic, local search, and other advanced technologies are realized in programming languages such as C++ and Python
Frequently asked questions
Information
Table of contents
- Cover image
- Title page
- Table of Contents
- Copyright
- Dedication
- List of figures
- List of tables
- List of algorithms
- List of listings
- About the authors
- Preface
- Part One: Fundamentals
- Chapter One: Introduction
- Chapter Two: Optimization problems
- Chapter Three: Traditional methods
- Chapter Four: Metaheuristic algorithms
- Chapter Five: Simulated annealing
- Chapter Six: Tabu search
- Chapter Seven: Genetic algorithm
- Chapter Eight: Ant colony optimization
- Chapter Nine: Particle swarm optimization
- Chapter Ten: Differential evolution
- Part Two: Advanced technologies
- Chapter Eleven: Solution encoding and initialization operator
- Chapter Twelve: Transition operator
- Chapter Thirteen: Evaluation and determination operators
- Chapter Fourteen: Parallel metaheuristic algorithm
- Chapter Fifteen: Hybrid metaheuristic and hyperheuristic algorithms
- Chapter Sixteen: Local search algorithm
- Chapter Seventeen: Pattern reduction
- Chapter Eighteen: Search economics
- Chapter Nineteen: Advanced applications
- Chapter Twenty: Conclusion and future research directions
- Appendix A: Interpretations and analyses of simulation results
- Appendix B: Implementation in Python
- References
- Index