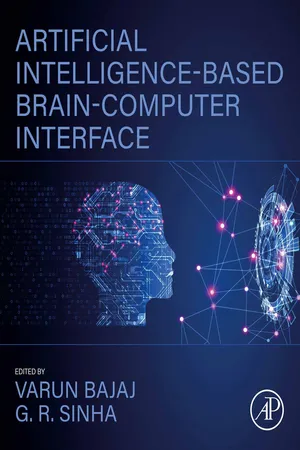
- 392 pages
- English
- ePUB (mobile friendly)
- Only available on web
Artificial Intelligence-Based Brain-Computer Interface
About This Book
Artificial Intelligence-Based Brain Computer Interface provides concepts of AI for the modeling of non-invasive modalities of medical signals such as EEG, MRI and FMRI. These modalities and their AI-based analysis are employed in BCI and related applications. The book emphasizes the real challenges in non-invasive input due to the complex nature of the human brain and for a variety of applications for analysis, classification and identification of different mental states. Each chapter starts with a description of a non-invasive input example and the need and motivation of the associated AI methods, along with discussions to connect the technology through BCI.
Major topics include different AI methods/techniques such as Deep Neural Networks and Machine Learning algorithms for different non-invasive modalities such as EEG, MRI, FMRI for improving the diagnosis and prognosis of numerous disorders of the nervous system, cardiovascular system, musculoskeletal system, respiratory system and various organs of the body. The book also covers applications of AI in the management of chronic conditions, databases, and in the delivery of health services.
- Provides readers with an understanding of key applications of Artificial Intelligence to Brain-Computer Interface for acquisition and modelling of non-invasive biomedical signal and image modalities for various conditions and disorders
- Integrates recent advancements of Artificial Intelligence to the evaluation of large amounts of clinical data for the early detection of disorders such as Epilepsy, Alcoholism, Sleep Apnea, motor-imagery tasks classification, and others
- Includes illustrative examples on how Artificial Intelligence can be applied to the Brain-Computer Interface, including a wide range of case studies in predicting and classification of neurological disorders
Frequently asked questions
Information
Table of contents
- Cover
- Title page
- Table of Contents
- Copyright
- Contributors
- 1: Multiclass sleep stage classification using artificial intelligence based time-frequency distribution and CNN
- 2: A comprehensive review of the movement imaginary brain-computer interface methods: Challenges and future directions
- 3: A new approach to feature extraction in MI-based BCI systems
- 4: Evaluation of power spectral and machine learning techniques for the development of subject-specific BCI
- 5: Concept of AI for acquisition and modeling of noninvasive modalities for BCI
- 6: Bi-LSTM-deep CNN for schizophrenia detection using MSST-spectral images of EEG signals
- 7: Detection of epileptic seizure disorder using EEG signals
- 8: Customized deep learning algorithm for drowsiness detection using single-channel EEG signal
- 9: EEG-based deep learning neural net for apnea detection
- 10: Classification of mental states from rational dilation wavelet transform and bagged tree classifier using EEG signals
- 11: A novel metaheuristic optimization method for robust spatial filter designation and classification of speech imagery tasks in EEG Brain-Computer Interface
- 12: Variational mode decomposition-based finger flexion detection using ECoG signals
- 13: An insight into the hardware and software aspects of a BCI system with focus on ultra-low power bulk driven OTA and Gm-C based filter design, and a detailed review of the recent AI/ML techniques
- 14: Deep autoencoder-based automated brain tumor detection from MRI data
- 15: Measure the superior functionality of machine intelligence in brain tumor disease prediction
- Index