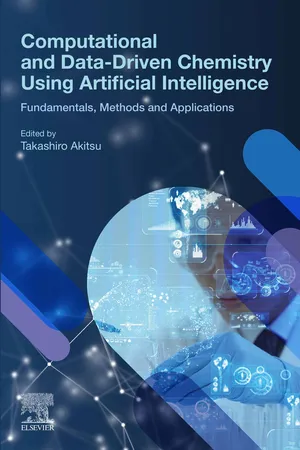
Computational and Data-Driven Chemistry Using Artificial Intelligence
Fundamentals, Methods and Applications
- 278 pages
- English
- ePUB (mobile friendly)
- Only available on web
Computational and Data-Driven Chemistry Using Artificial Intelligence
Fundamentals, Methods and Applications
About This Book
Computational and Data-Driven Chemistry Using Artificial Intelligence: Volume 1: Fundamentals, Methods and Applications highlights fundamental knowledge and current developments in the field, giving readers insight into how these tools can be harnessed to enhance their own work. Offering the ability to process large or complex data-sets, compare molecular characteristics and behaviors, and help researchers design or identify new structures, Artificial Intelligence (AI) holds huge potential to revolutionize the future of chemistry. Volume 1 explores the fundamental knowledge and current methods being used to apply AI across a whole host of chemistry applications.
Drawing on the knowledge of its expert team of global contributors, the book offers fascinating insight into this rapidly developing field and serves as a great resource for all those interested in exploring the opportunities afforded by the intersection of chemistry and AI in their own work. Part 1 provides foundational information on AI in chemistry, with an introduction to the field and guidance on database usage and statistical analysis to help support newcomers to the field. Part 2 then goes on to discuss approaches currently used to address problems in broad areas such as computational and theoretical chemistry; materials, synthetic and medicinal chemistry; crystallography, analytical chemistry, and spectroscopy. Finally, potential future trends in the field are discussed.
- Provides an accessible introduction to the current state and future possibilities for AI in chemistry
- Explores how computational chemistry methods and approaches can both enhance and be enhanced by AI
- Highlights the interdisciplinary and broad applicability of AI tools across a wide range of chemistry fields
Frequently asked questions
Information
Table of contents
- Cover image
- Title page
- Table of Contents
- Copyright
- Contributors
- About the editor
- Preface
- Chapter 1: Introductory chapter
- Chapter 2: Goal-directed generation of new molecules by AI methods
- Chapter 3: Salen-type metal complexes based on structural database of X-ray crystallography
- Chapter 4: Approaches using AI in medicinal chemistry
- Chapter 5: Application of machine learning algorithms for use in material chemistry
- Chapter 6: Predicting conformers of flexible metal complexes using deep neural network
- Chapter 7: Predicting activity and activation factor of catalytic reactions using machine learning
- Chapter 8: Convolutional neural networks for the design and analysis of nonfullerene acceptors
- Index