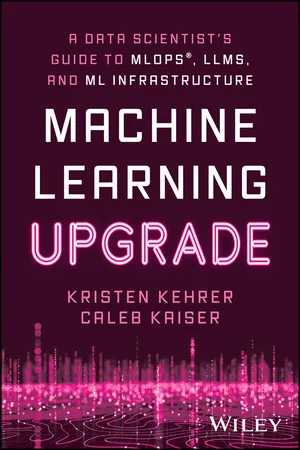
Machine Learning Upgrade: A Data Scientist's Guide to MLOps, LLMs, and ML Infrastructure
A Data Scientist's Guide to MLOps, LLMs, and ML Infrastructure
- English
- ePUB (mobile friendly)
- Only available on web
Machine Learning Upgrade: A Data Scientist's Guide to MLOps, LLMs, and ML Infrastructure
A Data Scientist's Guide to MLOps, LLMs, and ML Infrastructure
About This Book
A much-needed guide to implementing new technology in workspaces
From experts in the field comes Machine Learning Upgrade: A Data Scientist's Guide to MLOps, LLMs, and ML Infrastructure, a book that provides data scientists and managers with best practices at the intersection of management, large language models (LLMs), machine learning, and data science. This groundbreaking book will change the way that you view the pipeline of data science. The authors provide an introduction to modern machine learning, showing you how it can be viewed as a holistic, end-to-end systemânot just shiny new gadget in an otherwise unchanged operational structure. By adopting a data-centric view of the world, you can begin to see unstructured data and LLMs as the foundation upon which you can build countless applications and business solutions. This book explores a whole world of decision making that hasn't been codified yet, enabling you to forge the future using emerging best practices.
- Gain an understanding of the intersection between large language models and unstructured data
- Follow the process of building an LLM-powered application while leveraging MLOps techniques such as data versioning and experiment tracking
- Discover best practices for training, fine tuning, and evaluating LLMs
- Integrate LLM applications within larger systems, monitor their performance, and retrain them on new data
This book is indispensable for data professionals and business leaders looking to understand LLMs and the entire data science pipeline.
Frequently asked questions
Information
Table of contents
- Cover
- Table of Contents
- Title Page
- Introduction
- Acknowledgments
- About the Authors
- About the Technical Editor
- Chapter 1: A Gentle Introduction to Modern Machine Learning
- Chapter 2: An End-to-End Approach
- Chapter 3: A Data-Centric View
- Chapter 4: Standing Up Your LLM
- Chapter 5: Putting Together an Application
- Chapter 6: Rounding Out the ML Life Cycle
- Chapter 7: Review of Best Practices
- Appendix: Additional LLM Example
- Index
- Copyright
- End User License Agreement