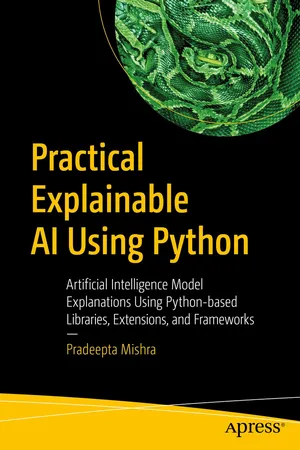
Practical Explainable AI Using Python
Artificial Intelligence Model Explanations Using Python-based Libraries, Extensions, and Frameworks
- English
- ePUB (mobile friendly)
- Only available on web
Practical Explainable AI Using Python
Artificial Intelligence Model Explanations Using Python-based Libraries, Extensions, and Frameworks
About This Book
Learn the ins and outs of decisions, biases, and reliability of AI algorithms and how to make sense of these predictions. This book explores the so-called black-box models to boost the adaptability, interpretability, and explainability of the decisions made by AI algorithms using frameworks such as Python XAI libraries, TensorFlow 2.0+, Keras, and custom frameworks using Python wrappers.
You'll begin with an introduction to model explainability and interpretability basics, ethical consideration, and biases in predictions generated by AI models. Next, you'll look at methods and systems to interpret linear, non-linear, and time-series models used in AI. The book will also cover topics ranging from interpreting to understanding how an AI algorithm makes a decision Further, you will learn the most complex ensemble models, explainability, and interpretability using frameworks such as Lime, SHAP, Skater, ELI5, etc. Moving forward, youwill be introduced to model explainability for unstructured data, classification problems, and natural language processingârelated tasks. Additionally, the book looks at counterfactual explanations for AI models. Practical Explainable AI Using Python shines the light on deep learning models, rule-based expert systems, and computer vision tasks using various XAI frameworks.
What You'll Learn
- Review the different ways of making an AI model interpretable and explainable
- Examine the biasness and good ethical practices of AI models
- Quantify, visualize, and estimate reliability of AI models
- Design frameworks to unbox the black-box models
- Assess the fairness of AI models
- Understand the building blocks of trust in AI models
- Increase the level of AI adoption
Who This Book Is For
AI engineers, data scientists, and software developers involved in driving AI projects/ AI products.
Frequently asked questions
Information
Table of contents
- Cover
- Front Matter
- 1. Model Explainability and Interpretability
- 2. AI Ethics, Biasness, and Reliability
- 3. Explainability for Linear Models
- 4. Explainability for Non-Linear Models
- 5. Explainability for Ensemble Models
- 6. Explainability for Time Series Models
- 7. Explainability for NLP
- 8. AI Model Fairness Using a What-If Scenario
- 9. Explainability for Deep Learning Models
- 10. Counterfactual Explanations for XAI Models
- 11. Contrastive Explanations for Machine Learning
- 12. Model-Agnostic Explanations by Identifying Prediction Invariance
- 13. Model Explainability for Rule-Based Expert Systems
- 14. Model Explainability for Computer Vision
- Back Matter