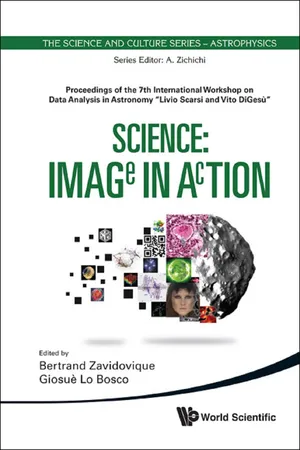
Science: Image in Action
Proceedings of the 7th International Workshop on Data Analysis in Astronomy "Livio Scarsi and Vito DiGesĂš"
- 328 pages
- English
- ePUB (mobile friendly)
- Available on iOS & Android
Science: Image in Action
Proceedings of the 7th International Workshop on Data Analysis in Astronomy "Livio Scarsi and Vito DiGesĂš"
About This Book
The book gathers articles that were exposed during the seventh edition of the Workshop “Data Analysis in Astronomy”. It illustrates a current trend to search for common expressions or models transcending usual disciplines, possibly associated with some lack in the Mathematics required to model complex systems. In that, data analysis would be at the epicentre and a key facilitator of some current integrative phase of Science.
It is all devoted to the question of “representation in Science”, whence its name, IMAGe IN AcTION, and main thrusts
-->
- Part A: Information: data organization and communication,
- Part B: System: structure and behaviour,
- Part C: Data — System representation.
-->
Such a classification makes concepts as “complexity” or “dynamics” appear like transverse notions: a measure among others or a dimensional feature among others.
Part A broadly discusses a dialogue between experiments and information, be information extracted-from or brought-to experiments. The concept is fundamental in statistics and tailors to the emergence of collective behaviours. Communication then asks for uncertainty considerations — noise, indeterminacy or approximation — and its wider impact on the couple perception-action. Clustering being all about uncertainty handling, data set representation appears not to be the only solution: Introducing hierarchies with adapted metrics, a priori pre-improving the data resolution are other methods in need of evaluation. The technology together with increasing semantics enables to involve synthetic data as simulation results for the multiplication of sources.
Part B plays with another couple important for complex systems: state vs. transition. State-first descriptions would characterize physics, while transition-first would fit biology. That could stem from life producing dynamical systems in essence. Uncertainty joining causality here, geometry can bring answers: stable patterns in the state space involve constraints from some dynamics consistency. Stable patterns of activity characterize biological systems too. In the living world, the complexity — i.e. a global measure on both states and transitions — increases with consciousness: this might be a principle of evolution. Beside geometry or measures, operators and topology have supporters for reporting on dynamical systems. Eventually targeting universality, the category theory of topological thermodynamics is proposed as a foundation of dynamical system understanding.
Part C details examples of actual data-system relations in regards to explicit applications and experiments. It shows how pure computer display and animation techniques link models and representations to “reality” in some “concrete” virtual, manner. Such techniques are inspired from artificial life, with no connection to physical, biological or physiological phenomena! The Virtual Observatory is the second illustration of the evidence that simulation helps Science not only in giving access to more flexible parameter variability, but also due to the associated data and method storing-capabilities. It fosters interoperability, statistics on bulky corpuses, efficient data mining possibly through the web etc. in short a reuse of resources in general, including novel ideas and competencies. Other examples deal more classically with inverse modelling and reconstruction, involving Bayesian techniques or chaos but also fractal and symmetry.
Contents:
- Information: Data Organization and Communication:
- Statistical Information: A Bayesian Perspective (R B Stern & C A de B Pereira)
- Multi-A(ge)nt Graph Patrolling and Partitioning (Y Elor & A M Bruckstein)
- The Role of Noise in Brain Function (S Roy & R Llinás)
- F-granulation, Generalized Rough Entropy and Image Analysis (S K Pal)
- Fast Redshift Clustering with the Baire (Ultra) Metric (F Murtagh & P Contreras)
- Interactive Classification Oriented Superresolution of Multispectral Images (P Ruiz, J V Talents, J Mateos, R Molina & A K Katsaggelos)
- Blind Processing in Astrophysical Data Analysis (E Salerno & L Bedini)
- The Extinction Map of the Orion Molecular Cloud (G Scandariato (Best Student's Paper), I Pagano & M Robberto)
- System: Structure and Behaviour:
- Common Grounds: The Role of Perception in Science and the Nature of Transitions (G Bernroider)
- Looking the World from Inside: Intrinsic Geometry of Complex Systems (L Boi)
- The Butterfly and the Photon: New Perspectives on Unpredictability, and the Notion of Casual Reality, in Quantum Physics (T N Palmer)
- Self-replicated Wave Patterns in Neural Networks with Complex Threshold (V I Nekorkin)
- A Local Explication of Causation (G Boniolo, R Faraldo & A Saggion)
- Evolving Complexity, Cognition, and Consciousness (H Liljenström)
- Self-Assembly, Modularity and Physical Complexity (S E Ahnert)
- The Category of Topological Thermodynamics (R M Kiehn)
- Anti-Phase Spiking Patterns (M P Igaev, A S Dmitrichev & V I Nekorkin)
- Data/System Representation:
- Reality, Models and Representations: The Case of Galaxies, Intelligence and Avatars (J-C Heudin)
- Astronomical Images and Data Mining in the International Virtual Observatory Context (F Pasian, M Brescia & G Longo)
- Dame: A Web Oriented Infrastructure for Scientific Data Mining and Exploration (S Cavuoti, M Brescia, G Longo, M Garofalo & A Nocella)
- Galactic Phase Spaces (D Chakrabarty)
- From Data to Images: A Shape Based Approach for Fluorescence Tomography (O Dorn & K E Prieto)
- The Influence of Texture Symmetry in Marker Pointing: Experimenting with Humans and Algorithms (M Cardaci & M E Tabacchi)
- A Multiscale Autocorrelation Function for Anisotropy Studies (M Scuderi, M De Domenico, A Insolia & H Lyberis)
- A Multiscale, Lacunarity and Neural Network Method for γ/h Discrimination in Extensive Air Showers (A Pagliaro, F D'anna, G D'alí Staiti)
- Bayesian Semi-Parametric Curve-Fitting and Clustering in SDSS Data (S Mukkhopadhyay, S Roy & S Bhattacharya)
Readership: Students, researchers and academics.
Frequently asked questions
Information

















Table of contents
- Cover Page
- Title Page
- Copyright Page
- Contents
- Workshop photograph
- Organizing Committees
- Sponsors
- Preface
- Memory of Vito Di GesĂş
- Part A Information: Data Organization and Communication
- Part B System: Structure and Behaviour
- Part C Data/System Representation
- Author Index
- Participants