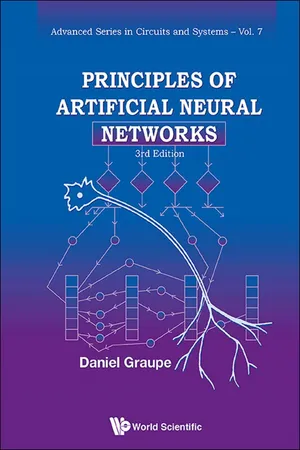
- 500 pages
- English
- ePUB (mobile friendly)
- Available on iOS & Android
Principles Of Artificial Neural Networks (3rd Edition)
About This Book
Artificial neural networks are most suitable for solving problems that are complex, ill-defined, highly nonlinear, of many and different variables, and/or stochastic. Such problems are abundant in medicine, in finance, in security and beyond.
This volume covers the basic theory and architecture of the major artificial neural networks. Uniquely, it presents 18 complete case studies of applications of neural networks in various fields, ranging from cell-shape classification to micro-trading in finance and to constellation recognition — all with their respective source codes. These case studies demonstrate to the readers in detail how such case studies are designed and executed and how their specific results are obtained.
The book is written for a one-semester graduate or senior-level undergraduate course on artificial neural networks. It is also intended to be a self-study and a reference text for scientists, engineers and for researchers in medicine, finance and data mining.
Contents:
- Introduction and Role of Artificial Neural Networks
- Fundamentals of Biological Neural Networks
- Basic Principles of ANNs and Their Early Structures
- The Perceptron
- The Madaline
- Back Propagation
- Hopfield Networks
- Counter Propagation
- Large Scale Memory Storage and Retrieval (LAMSTAR) Network
- Adaptive Resonance Theory
- The Cognitron and the Neocognitron
- Statistical Training
- Recurrent (Time Cycling) Back Propagation Networks
Readership: Graduate and advanced senior students in artificial intelligence, pattern recognition & image analysis, neural networks, computational economics and finance, and biomedical engineering.
Frequently asked questions
Information
Table of contents
- Front Cover
- Half Title
- Author Title
- Title Page
- Copyright
- Dedication
- Contents
- Acknowledgments
- Preface to the Third Edition
- Preface to the Second Edition
- Preface to the First Edition
- Chapter 1. Introduction and Role of Artificial Neural Networks
- Chapter 2. Fundamentals of Biological Neural Networks
- Chapter 3. Basic Principles of ANNs and Their Early Structures
- Chapter 4. The Perceptron
- Chapter 5. The Madaline
- Chapter 6. Back Propagation
- Chapter 7. Hopfield Networks
- Chapter 8. Counter Propagation
- Chapter 9. Large Scale Memory Storage and Retrieval (LAMSTAR) Network
- Chapter 10. Adaptive Resonance Theory
- Chapter 11. The Cognitron and the Neocognitron
- Chapter 12. Statistical Training
- Chapter 13. Recurrent (Time Cycling) Back Propagation Networks
- Problems
- References
- Author Index
- Subject Index