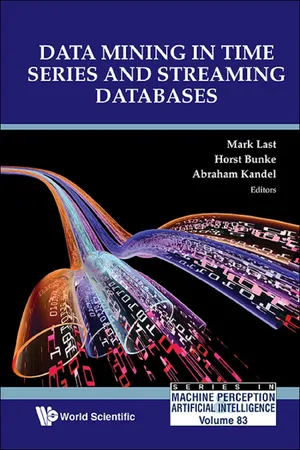
- 196 pages
- English
- ePUB (mobile friendly)
- Available on iOS & Android
Data Mining in Time Series and Streaming Databases
About This Book
-->
This compendium is a completely revised version of an earlier book, Data Mining in Time Series Databases, by the same editors. It provides a unique collection of new articles written by leading experts that account for the latest developments in the field of time series and data stream mining.
The emerging topics covered by the book include weightless neural modeling for mining data streams, using ensemble classifiers for imbalanced and evolving data streams, document stream mining with active learning, and many more. In particular, it addresses the domain of streaming data, which has recently become one of the emerging topics in Data Science, Big Data, and related areas. Existing titles do not provide sufficient information on this topic.
--> Contents:
- Streaming Data Mining with Massive Online Analytics (MOA) (Albert Bifet, Jesse Read, Geoff Holmes and Bernhard Pfahringer)
- Weightless Neural Modeling for Mining Data Streams (Douglas O Cardoso, João Gama and Felipe França)
- Ensemble Classifiers for Imbalanced and Evolving Data Streams (Dariusz Brzezinski and Jerzy Stefanowski)
- Consensus Learning for Sequence Data (Andreas Nienkötter and Xiaoyi Jiang)
- Clustering-Based Classification of Document Streams with Active Learning (Mark Last, Maxim Stoliar and Menahem Friedman)
- Supporting the Mining of Big Data by Means of Domain Knowledge During the Pre-mining Phases (Rémon Cornelisse and Sunil Choenni)
- Data Analytics: Industrial Perspective & Solutions for Streaming Data (Mohsin Munir, Sebastian Baumbach, Ying Gu, Andreas Dengel and Sheraz Ahmed)
-->
--> Readership: Researchers, academics, professionals and graduate students in artificial intelligence, machine learning, databases, and information science. -->
Keywords:Time Series;Data Streams;Big Data;Internet of Things;Concept Drift;Sequence Mining;Episode Mining;Incremental Learning;Active LearningReview:0
Frequently asked questions
Information
Table of contents
- Cover
- Halftitle
- Title
- Copyright
- Dedication
- Preface
- About the Editors
- About the Contributors
- Contents
- Chapter 1. Streaming Data Mining with Massive Online Analytics (MOA)
- Chapter 2. Weightless Neural Modeling for Mining Data Streams
- Chapter 3. Ensemble Classifiers for Imbalanced and Evolving Data Streams
- Chapter 4. Consensus Learning for Sequence Data
- Chapter 5. Clustering-Based Classification of Document Streams with Active Learning
- Chapter 6. Supporting the Mining of Big Data by Means of Domain Knowledge During the Pre-mining Phases
- Chapter 7. Data Analytics: Industrial Perspective & Solutions for Streaming Data
- Index