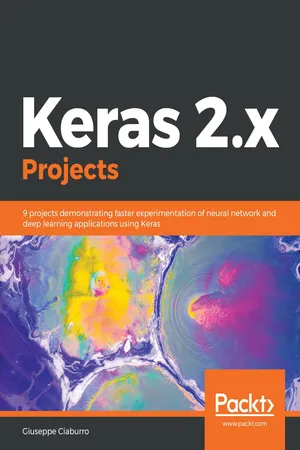
Keras 2.x Projects
9 projects demonstrating faster experimentation of neural network and deep learning applications using Keras
Giuseppe Ciaburro
- 394 pages
- English
- ePUB (mobile friendly)
- Available on iOS & Android
Keras 2.x Projects
9 projects demonstrating faster experimentation of neural network and deep learning applications using Keras
Giuseppe Ciaburro
About This Book
Demonstrate fundamentals of Deep Learning and neural network methodologies using Keras 2.x
Key Features
- Experimental projects showcasing the implementation of high-performance deep learning models with Keras.
- Use-cases across reinforcement learning, natural language processing, GANs and computer vision.
- Build strong fundamentals of Keras in the area of deep learning and artificial intelligence.
Book Description
Keras 2.x Projects explains how to leverage the power of Keras to build and train state-of-the-art deep learning models through a series of practical projects that look at a range of real-world application areas.
To begin with, you will quickly set up a deep learning environment by installing the Keras library. Through each of the projects, you will explore and learn the advanced concepts of deep learning and will learn how to compute and run your deep learning models using the advanced offerings of Keras. You will train fully-connected multilayer networks, convolutional neural networks, recurrent neural networks, autoencoders and generative adversarial networks using real-world training datasets. The projects you will undertake are all based on real-world scenarios of all complexity levels, covering topics such as language recognition, stock volatility, energy consumption prediction, faster object classification for self-driving vehicles, and more.
By the end of this book, you will be well versed with deep learning and its implementation with Keras. You will have all the knowledge you need to train your own deep learning models to solve different kinds of problems.
What you will learn
- Apply regression methods to your data and understand how the regression algorithm works
- Understand the basic concepts of classification methods and how to implement them in the Keras environment
- Import and organize data for neural network classification analysis
- Learn about the role of rectified linear units in the Keras network architecture
- Implement a recurrent neural network to classify the sentiment of sentences from movie reviews
- Set the embedding layer and the tensor sizes of a network
Who this book is for
If you are a data scientist, machine learning engineer, deep learning practitioner or an AI engineer who wants to build speedy intelligent applications with minimal lines of codes, then this book is the best fit for you. Sound knowledge of machine learning and basic familiarity with Keras library would be useful.
Frequently asked questions
Information
Reuters Newswire Topics Classifier in Keras
- Natural language processing
- The Natural Language Toolkit
- Corpora
- Word and sentence tokenize
- Implementing a DNN to label sentences
Natural language processing
- Natural language analysis (NLA): This aims to study the understanding of natural language by machines. Deals with the reduction of a text in natural language in a formal and univocal representation.
- Natural language generation (NLG): This aims to study how machines can generate sentences in natural language. It deals with the construction of applications capable of producing correct sentences in a specific language.
NLP phases
- Morphology analysis
- Syntax analysis
- Semantic analysis
- Pragmatic analysis
Morphology analysis
Syntax analysis
Table of contents
- Title Page
- Copyright and Credits
- About Packt
- Contributors
- Preface
- Getting Started with Keras
- Modeling Real Estate Using Regression Analysis
- Heart Disease Classification with Neural Networks
- Concrete Quality Prediction Using Deep Neural Networks
- Fashion Article Recognition Using Convolutional Neural Networks
- Movie Reviews Sentiment Analysis Using Recurrent Neural Networks
- Stock Volatility Forecasting Using Long Short-Term Memory
- Reconstruction of Handwritten Digit Images Using Autoencoders
- Robot Control System Using Deep Reinforcement Learning
- Reuters Newswire Topics Classifier in Keras
- What is Next?
- Other Books You May Enjoy