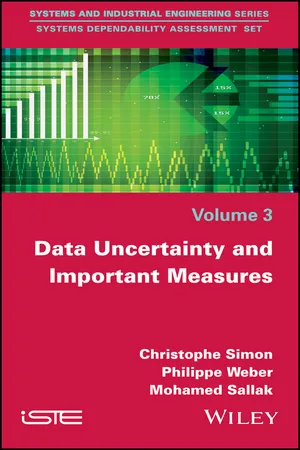
This is a test
- English
- ePUB (mobile friendly)
- Available on iOS & Android
eBook - ePub
Data Uncertainty and Important Measures
Book details
Book preview
Table of contents
Citations
About This Book
The first part of the book defines the concept of uncertainties and the mathematical frameworks that will be used for uncertainty modeling. The application to system reliability assessment illustrates the concept. In the second part, evidential networks as a new tool to model uncertainty in reliability and risk analysis is proposed and described. Then it is applied on SIS performance assessment and in risk analysis of a heat sink. In the third part, Bayesian and evidential networks are used to deal with important measures evaluation in the context of uncertainties.
Frequently asked questions
At the moment all of our mobile-responsive ePub books are available to download via the app. Most of our PDFs are also available to download and we're working on making the final remaining ones downloadable now. Learn more here.
Both plans give you full access to the library and all of Perlego’s features. The only differences are the price and subscription period: With the annual plan you’ll save around 30% compared to 12 months on the monthly plan.
We are an online textbook subscription service, where you can get access to an entire online library for less than the price of a single book per month. With over 1 million books across 1000+ topics, we’ve got you covered! Learn more here.
Look out for the read-aloud symbol on your next book to see if you can listen to it. The read-aloud tool reads text aloud for you, highlighting the text as it is being read. You can pause it, speed it up and slow it down. Learn more here.
Yes, you can access Data Uncertainty and Important Measures by Christophe Simon, Philippe Weber, Mohamed Sallak in PDF and/or ePUB format, as well as other popular books in Mathématiques & Mathématiques générales. We have over one million books available in our catalogue for you to explore.
Information
1
Why and Where Uncertainties
This book shows our work in the School of Nancy on taking into account several types of uncertainty in the assessment of dependability parameters. For this purpose, we are modeling uncertainties through additive and nonadditive theories for modeling epistemic and aleatory uncertainties. Several theories are used for this purpose in this book.
An important problem in reliability theory is to identify components within the system that significantly influence system behavior with respect to reliability or availability. Because all components cannot be improved at once to improve the system reliability, priority should be given to components that are more important. The importance measures have been developed to analyze the impact and influence of some parameters, components or group of components on the global performance of a system. The components concerned are those acting effectively to improve the system performances, or those on which to release or to impose requirements to meet or to maintain an expected level of performance. The assessment of these measures is associated with the probabilities of the system functioning (or malfunctioning) according to the state of the components. In dependability analysis, they can be used to identify the critical components, mincuts, etc., or more generally influence measures on the reliability, the availability or the maintainability of the system.
1.1. Sources and forms of uncertainty
Usually, knowledge can be defined by several characteristics such as its type and its source [DUB 10]. Based on this classification, knowledge can be generic, singular or coming from beliefs (Table 1.1). In addition, it comes from either historical-based or observation-based sources (Table 1.2).
Table 1.1. Types of knowledge according to [DUB 10]
Generic knowledge | Repeated observations as dependence rules between variables or influence links |
Singular evidence | Singular situations like inspection results, test results or measurements |
Beliefs | Unobserved singular events as extreme phenomenon or unrealized actions |
Table 1.2. Knowledge sources according to [DUB 10]
Historical | Classes of situations (physical laws, statistical knowledge, etc.) |
Observations | Particular situations known as true (measurements, results of tests, etc.) |
Moreover, knowledge can be classified from other characteristics as their nature or the expression mode (Table 1.3).
Table 1.3. Other characteristics of knowledge
Nature | Knowledge can be expressed subjectively (individual and subject to change according to people) or objectively (no personal factor in the judgment provided) |
Expression | Knowledge can be qualitative (order, preference, etc.) or quantitative (scalar values, intervals with or without information, probability distribution, etc.) |
Whereas generic knowledge and singular evidences are based on observed (or observable) events, beliefs are based on unmeasured (or unmeasurable) events. Therefore, beliefs are potentially more difficult to express and can be considered more complex in terms of uncertainty. Moreover, the subjective or objective nature of knowledge implies the modes and shape of different expressions according to their dependence on the personality and the level of knowledge possessed by people or experts.
Finally, the qualitative or quantitative character of knowledge can give several kinds of expressions which are more or less precise (order, preferences, scalar values, intervals, etc.). In conclusion, the different characteristics of knowledge induce several levels of (im)precision in their expression. These levels induce uncertainties on knowledge which are mainly characterized by their sources and types.
1.2. Types of uncertainty
Many works concern the classification of uncertainties [HOF 94, FER 96, HEL 97, RIE 12]. Generally, the taxonomy of uncertainty is done with two distinct categories: aleatory or epistemic.
- – Aleatory uncertainty is due to the random character or the natural variability of physical phenomena (the values are precise but different according to natural variations). Some researchers talk of stochastic or variability uncertainty. This uncertainty is usually due to measurable elements [WIN 96], and it is considered irreducible because it is only due to the natural variations of physical phenomenon [BAE 04]. Aleatory uncertainty is usually associated with objective knowledge coming from generic knowledge or singular observations.
- – Epistemic uncertainty is due to the imprecise character of knowledge or associated with the lack of knowledge. It is usually associated with non-measurable quantities [WIN 96] and it is considered as reducible since new information can reduce or eliminate this type of uncertainty. It is mainly encountered with subjective data based on beliefs and can be quantitative or qualitative.
1.3. Sources of uncertainty
An important question comes from the sources of uncertainty. These sources are our own inability to know the exact values or state of the system and its components in the dependability point of view. This inability can be technical or conceptual. For instance, Pate-Cornell [COR 96] used six levels of uncertainty to obtain a family of risk curves in the presence of both aleatory and epistemic uncertainties. Smithson [SMI 89] proposed a taxonomy of ignorance (see Figure 1.1). In his work, ignorance is considered multiple and at several levels. Ignorance is the top level concept of his taxonomy. Some parts of this taxonomy concern irrelevance of knowledge but they are outside the scope of our work. The second part concerns error and is well developed but less clear for our purpose.
We can also add to this list of knowledge imperfection the notion of inconsistency which appears when knowledge is formulated by one or several sources that provide contradictory information [OSE 03].
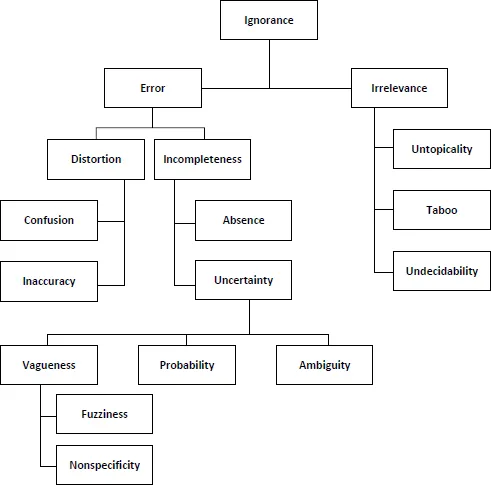
Figure 1.1. Taxonomy of ignorance
For our purpose of numerical assessment of risk and dependability, we prefer the taxonomy proposed by Fisher [FIS 99] which is a particular point of view of the Smithson taxonomy (see Figure 1.2). This taxonomy seems more convenient and refers to a current meaning, for instance, developed in the special issue of Reliability Engineering & System Safety [HEL 04].
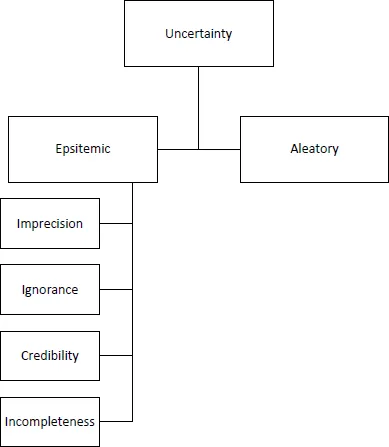
Figure 1.2. The taxonomy of uncertainty considered
Aleatory or random uncertainty has its roots in the natural...
Table of contents
- Cover
- Table of Contents
- Title
- Copyright
- Foreword
- Acknowledgments
- 1 Why and Where Uncertainties
- 2 Models and Language of Uncertainty
- 3 Risk Graphs and Risk Matrices: Application of Fuzzy Sets and Belief Reasoning
- 4 Dependability Assessment Considering Interval-valued Probabilities
- 5 Evidential Networks
- 6 Reliability Uncertainty and Importance Factors
- Conclusion
- Bibliography
- Index
- End User License Agreement